Lab 12: Markov Chain Monte Carlo algorithm#
\((x_{1},\ldots,x_{n})\) is a random sample generated from the exponential distribution with rate \(\lambda\). The prior of \(\lambda\) is the exponential distribution with mean 1/2. The posterior distribution of \(\lambda\) given \((x_{1},\ldots,x_{n})\) is
This is a gamma distribution with \(\alpha = n + 1\) and \(\beta = \sum_{i = 1}^{n}x_{i} + 2\). The posterior mean is \(\frac{\alpha}{\beta} = \frac{n + 1}{\sum_{i = 1}^{n}x_{i} + 2}\). Thus, the Bayesian estimate of \(\lambda\) is \(\frac{n + 1}{\sum_{i = 1}^{n}x_{i} + 2}\).
Suppose the data is (1.001, 0.065, 0.014, 1.601, 0.288, 0.095, 0.401, 0.227, 0.234, 0.488). Then, the Bayesian estimate of \(\lambda\) is
Here, we use the MCMC algorithm to approximate the posterior distribution \(f\left( \lambda \middle| X \right)\), then calculate the posterior mean.
The R code of the MCMC algorithm should have the following functions (1) Likelihood function, (2) Prior, (3) a function for updating \(\lambda\), and (4) a function for accepting or rejecting the proposed \(\lambda_{new}\).
########################################################
# mcmc algorithm: likelihood: exp, prior: exp
########################################################
loglikelihood <- function (x, lambda) {
n <- length(x)
loglike <- n*log(lambda) - sum(x) * lambda
return (loglike)
}
logprior <- function(lambda, theta) {
logprior <- log(theta) - lambda*theta
return (logprior)
}
update_lambda<-function(lambda, window_width){
newlambda <- lambda + (2*runif(1)-1) * window_width
return (newlambda)
}
#######################################################
# algorithm
########################################################
x = rexp(100)
samplesize = length(x)
theta <- 10 #prior of lambda
lambda_theory = (samplesize+1)/(sum(x)+theta)
totalround <- 100000
lambda <- 1:totalround
loglike <- 1:totalround
window_width <- 0.05
oldlambda <- 1 #initial value of lambda
oldloglike <- loglikelihood(x, oldlambda)
oldlogprior <- logprior(oldlambda, theta)
for (i in 1:totalround)
{
newlambda <- update_lambda(oldlambda, window_width)
if (newlambda<0) newlambda = -newlambda
newloglike <- loglikelihood(x, newlambda)
newlogprior <- logprior(newlambda, theta)
hastings_ratio <- min(exp((newloglike+newlogprior)-(oldloglike+oldlogprior)),1)
if(runif(1) < hastings_ratio){
lambda[i] = newlambda
loglike[i] = newloglike
oldlambda = newlambda
oldlogprior = newlogprior
}else{
lambda[i] = oldlambda
loglike[i] = oldloglike
}
}
plot(loglike,type="l")
burnin = totalround/2
print(paste("MCMC estimate of lambda:", mean(lambda[burnin:totalround])))
print(paste("Bayesian estimate of lambda:",lambda_theory))
[1] "MCMC estimate of lambda: 0.829400187753933"
[1] "Bayesian estimate of lambda: 0.80551467738495"
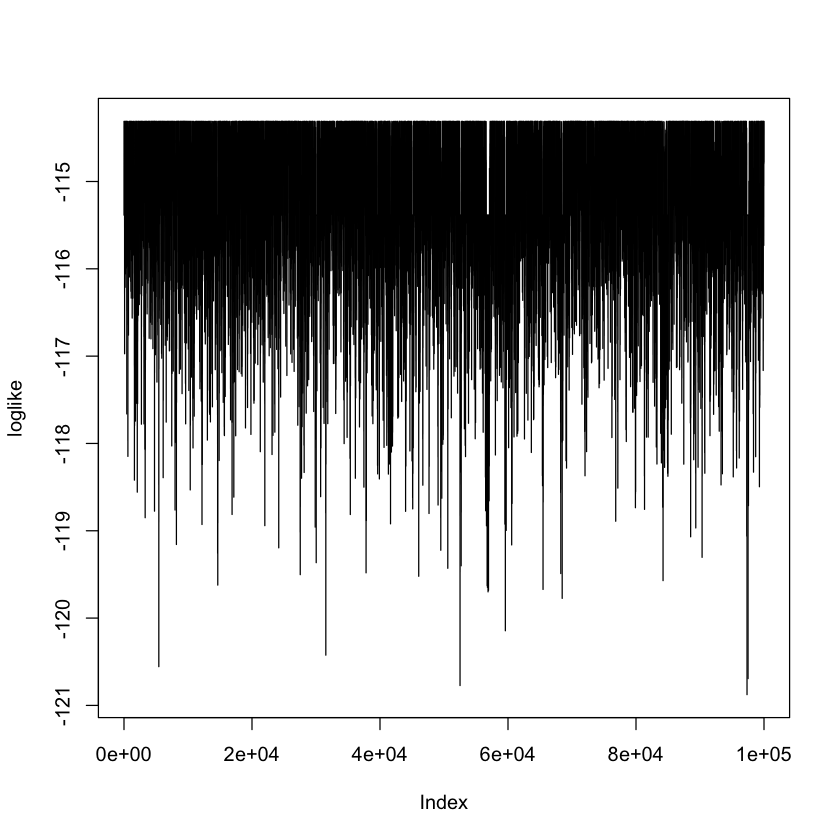
Bayesian phylogenetic inference#
Preparation: Download MrBayes at http://nbisweden.github.io/MrBayes/download.html Then, unzip the downloaded file MrBayes-3.2.7a.zip and navigate into the unzipped folder by typing cd ./MrBayes-3.2.7a in the terminal window.
To install MrBayes on your laptop, type ./configure and then type make. The executable mb is located in the src folder. To run MrBayes interactively, type ./mb. Alternatively, you may run MrBayes using a batch file.
The data file finch.nex is available in the example folder. We will run MrBayes on this data set. We first add a MrBayes block in the data file and then run MrBayes by the command line
../src/mb -i finch.nex